Meta begins testing its first in-house AI training chip
The push to develop in-house chips is part of a long-term plan in the meta, which is to bring down the cost of its huge infrastructure as the company puts expensive bets on AI devices to run development.
Listen to the story
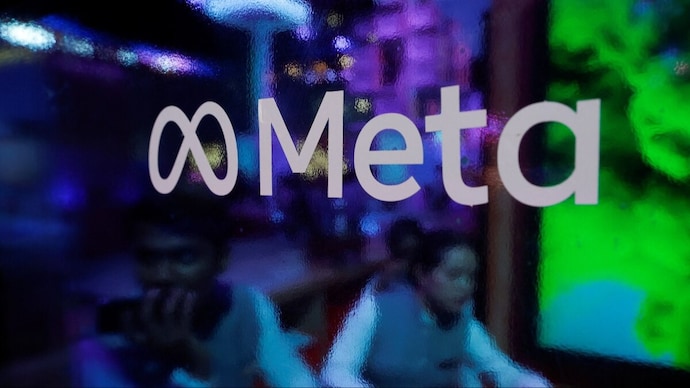
Facebook owner Meta is testing its first in-house chip to train the Artificial Intelligence System, a major milestone as it proceeds to designing its own custom silicon and reducing dependence on external suppliers such as NVDia, two sources told royters.
Sources said that the world’s largest social media company has started a small deployment of the chip and if the test is done well, there is a plan to increase production for widespread use.
The push to develop in-house chips is part of a long-term plan in the meta, which is to bring down the cost of its huge infrastructure as the company puts expensive bets on AI devices to run development.
Meta, which also owns Instagram and WhatsApp, has estimated a total of $ 2025 to $ 114 billion to $ 119 billion, including a large -scale AI infrastructure spent on the capital expenditure operating by $ 65 billion.
One of the sources said that the new training chip of the meta is a dedicated accelerator, which means that it is designed to handle only AI-specific tasks. This can make it more power-efficient than integrated graphics processing units (GPU) that is usually used for AI workloads.
The person is working with TSMC -based chip maker TSMC to produce a meta chip. Another source said that the first “tape-out” of the test-finished chip began after finishing the first “tape-out” of the chip, an important marker of success in the silicon development work that includes sending an initial design through the chip factory.
A specific tape-out costs ten million dollars and takes about three to six months to complete, due to no guarantee, the test will not be successful. A failure will require meta to diagnose the problem and repeat the tape-out stage.
Meta and TSMC refused to comment.
Chip is the latest in the company’s meta training and estimates accelerator (MTIA) series. The program has started a wobbly in the program and has scattered a chip in the same phase of development at one point.
However, Meta began to start using an MTIA chip last year, or users interact with it as a procedure involved in running the AI system, for recommended systems that determine which materials show on Facebook and Instagram News Feed.
Meta officials have stated that they want to start using their own chips by 2026 for training, or how to do this to “teach” the calculation-intensity process of feeding the AI system Reems.
Officials said that with the conclusion chip, the target for training chip is to start with recommended systems and later it is used for generic AI products such as chatboats Meta AI.
Meta Chief Product Officer Chris Cox said in Morgan Stanley Technology, Media and Telecom Conference last week, “We are working on how we will train for the recommended system and then how will we think about training and estimates for General AI finally.”
Cox described the Meta’s chip development efforts as “walk, crawl, run status”, but said that the authorities considered the first generation estimates for the recommendations to be a “big success”.
Meta first pulled the plug on the in-house custom invention chip, as it flopped into a small scale test-finance, which is now for the training chip, instead to reverse the course and to order billions of dollars worth billions of dollars of NVDia GPU in 2022.
The social media company has been one of the largest customers in NVIDIA since then collects an arsenal of GPU to train its models, including recommendations and ads systems and LLAMA Foundation Model Series. Units also guess for more than 3 billion people who use its app each day.
The value of those GPUs has been thrown into the question this year as AI researchers have rapidly expressed doubt that how much progress can be made by releasing “scale up” by adding more data and computing power to the big language model.
Those doubts were reinforced with the late January launch of the new low -cost model from the Chinese Startup Deepsek, which optimize computational efficiency by depending on most of the most dependent models.
In a deep-inspired global route in AI shares, Nvidia’s shares lost the fifth of their value at one point. Later he re -acquired most of the grounds in which investors who tease the company’s chips will remain the industry standards for training and estimates, although they have a drop.Paid again on comprehensive business concerns.